One of my favorite books for quantitative investing is Machine Learning for Algorithmic Trading. However, a lot of these concepts might be difficult for someone who getting started in programming strategies. So I created this Machine Learning for Algorithmic Trading GPT which can answer any questions related to this book. While these aren’t perfect responses they will typically put you on the right path with a little input.
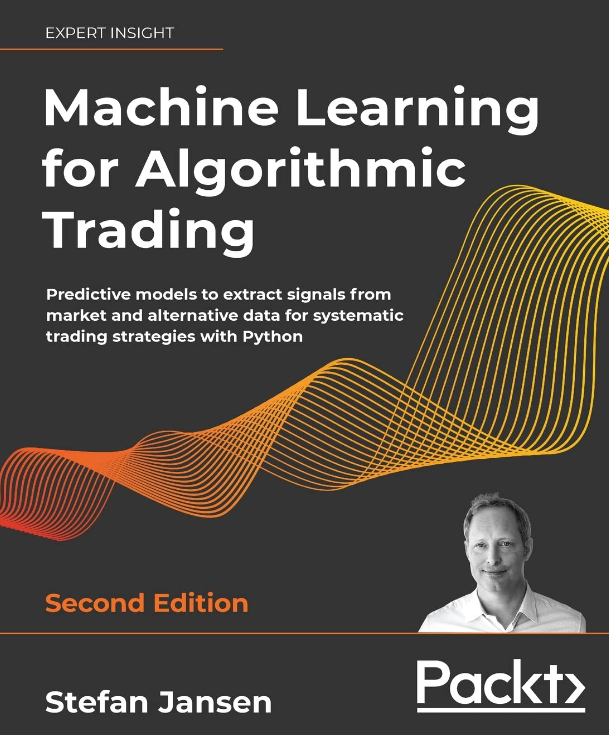
Sample Excerpt:
You:
Based on the references in this book where can I download stock data?
Machine Learning for Trading Tutor:
Based on the references in the book, here are the sources where you can download stock data:
- Quandl: Quandl provides stock price datasets covering the US equity markets for the years 2000-2018. The book mentions loading these datasets using pandas for analysis.
- yfinance: yfinance offers a way to download historical market data from Yahoo! Finance. This library, initially named fix-yahoo-finance, allows for the downloading of various data points including end-of-day and intraday prices. The book describes the process of using yfinance to download historical prices for various periods, at different frequencies, and adjusted OHLCV (Open, High, Low, Close, Volume) data at a one-minute frequency along with corporate actions.
- AlgoSeek: For more detailed intraday data, AlgoSeek provides adjustment factors to correct pricing and volumes for stock splits, dividends, and other corporate actions. The book suggests AlgoSeek as a source for such data, emphasizing its use for accurate market data analysis.
- SEC (U.S. Securities and Exchange Commission): While not directly a source of stock prices, the SEC’s EDGAR database can be used to download financial statements and related information, which can be valuable for fundamental analysis. The book provides guidance on using Python to extract data from EDGAR.
- Pandas DataReader: For additional fundamental data sources, the book mentions that many are accessible using the pandas_datareader module, which facilitates the download of financial data from a variety of sources including Yahoo Finance, Google Finance, and others.
- International equity data and macro fundamental data: Besides U.S. stock data, the book also references sources for international equity data like Stooq and various macro fundamental and benchmark data from the Federal Reserve, indicating the broad scope of data sources available for trading and analysis.
These sources provide a comprehensive toolkit for accessing both historical and real-time market data, fundamental data, and other financial information crucial for trading and investment analysis.
You:
Can you write me a Python function for each of these with sample code to download data from this source.
Machine Learning for Trading Tutor
Sure, I’ll provide you with Python functions for downloading stock data from each of the mentioned sources. Please note that these examples require you to have the respective libraries installed and, in some cases, to register for API keys where necessary.
1. Quandl
For Quandl, you’ll need to install the quandl
Python package and obtain an API key from the Quandl website.
pythonCopy code
import quandl
def download_quandl_data(symbol, start_date, end_date, api_key):
quandl.ApiConfig.api_key = api_key
data = quandl.get(symbol, start_date=start_date, end_date=end_date)
return data
# Example usage
# Replace 'YOUR_API_KEY' with your actual Quandl API key
# data = download_quandl_data('WIKI/AAPL', '2020-01-01', '2020-12-31', 'YOUR_API_KEY')
2. Yahoo! Finance
For Yahoo! Finance, use the yfinance
package. This doesn’t require an API key.
pythonCopy code
import yfinance as yf
def download_yfinance_data(symbol, start_date, end_date):
data = yf.download(symbol, start=start_date, end=end_date)
return data
# Example usage
# data = download_yfinance_data('AAPL', '2020-01-01', '2020-12-31')
3. AlgoSeek
Downloading data from AlgoSeek would typically require accessing their proprietary database or API, for which you might need an account or subscription. Here’s a generic approach assuming you have access:
# Placeholder for AlgoSeek data downloading
# Please refer to AlgoSeek's documentation and your subscription details for specific access methods
# Placeholder for AlgoSeek data downloading # Please refer to AlgoSeek's documentation and your subscription details for specific access methods
4. pandas_datareader
To use pandas_datareader
for downloading stock data, ensure you have this package installed.
import pandas_datareader.data as web
def download_pandas_datareader_data(symbol, start_date, end_date):
data = web.DataReader(symbol, 'yahoo', start_date, end_date)
return data
# Example usage
# data = download_pandas_datareader_data('AAPL', '2020-01-01', '2020-12-31')
5. SEC Filings (EDGAR)
Downloading data from the SEC’s EDGAR system for fundamental analysis typically involves parsing XBRL filings. This is more complex and requires specific libraries (e.g., python-edgar
or similar). Here’s a basic structure:
pythonCopy code
# Placeholder for EDGAR data downloading
# This operation is complex and requires parsing XBRL files; refer to specialized libraries and SEC's documentation
These examples are meant to get you started with downloading stock data from various sources. Depending on your specific needs and the details of your project, you might need to adjust parameters, handle API limits, or process the data further after downloading it.