I started off wanting to analyze the sustainability of mortgage buydowns by home builders. But as it happens, my ADHD had other plans. Three weeks later, here we are with code that looks for trends and anomalies in corporate financials. The code generates detailed HTML profiles for in-depth financial analysis of stocks.
In a series of previous posts, I’ve explained the extraction of corporate financial data and stock price data. Now, let’s go a step further by utilizing Plotly and Facebook’s Prophet library to predict these financial metrics and stock prices. It’s crucial to note that the goal here isn’t to necessarily predict future values but to model the data in such a way that we can quickly spot any anomalies. In this tutorial, we’ll be extracting data from HDF5 files, which were created in previous posts, so make sure to check those out first.
Generated HTML Output: A Preview of What the Code Produces
The code generates an in-depth profile of any stock specified. In this example, we take a look at Google. The output is a structured HTML file that includes a variety of information sections. First, it provides basic details such as the company’s name, ticker symbol, and the date of the most recent update. Next, it lists essential contact information, including the company’s web URL, phone number, and physical address. The sector and industry in which the company operates, as well as the number of full-time employees, are also displayed. The output then delves into valuation metrics like P/E ratios, enterprise value, and other financial ratios. Finally, it highlights key financial data points, such as market capitalization, EBITDA, and various earnings and revenue estimates. Overall, the code produces a multi-faceted profile that serves as a valuable resource for anyone looking to understand Alphabet Inc’s business and financial standing in great detail.
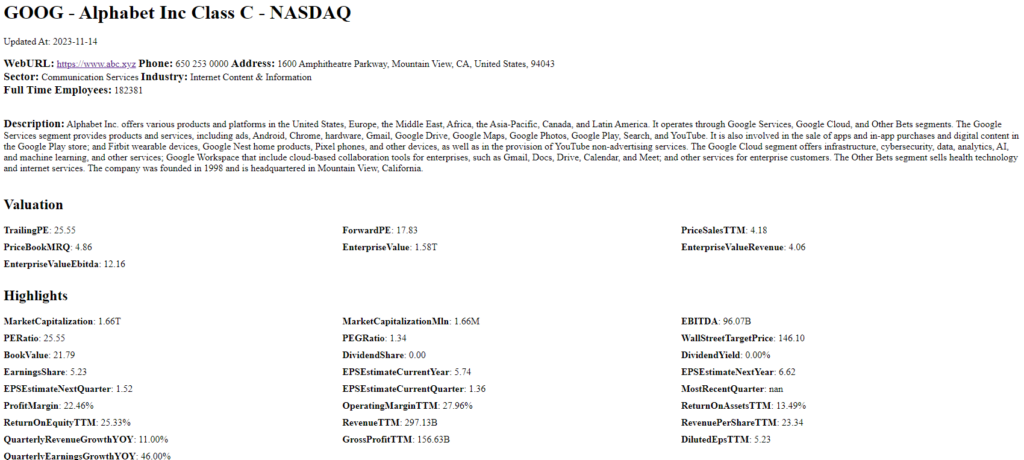
Forecasting Future Adjusted Close Prices with Facebook Prophet: A One-Year Outlook

This plot visualizes the historical and predicted adjusted close prices for a particular asset, utilizing the Facebook Prophet algorithm for the forecast. The x-axis represents the timeline, extending from the earliest available historical data to one year into the future. The y-axis shows the adjusted close prices. Historical prices are plotted as solid purple lines. The predicted value is a solid green line with blue dotted lines as the high and low predictions. This predictive model leverages the power of Facebook Prophet to analyze seasonal and trend components in the historical data, providing a one-year outlook on potential price movements.
Forecasting Future Financial Ratios with Facebook Prophet: A One-Year Outlook
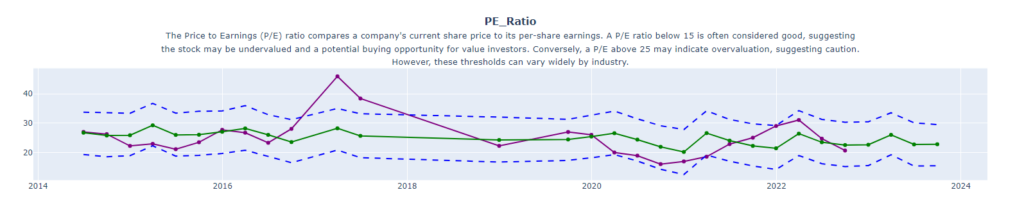
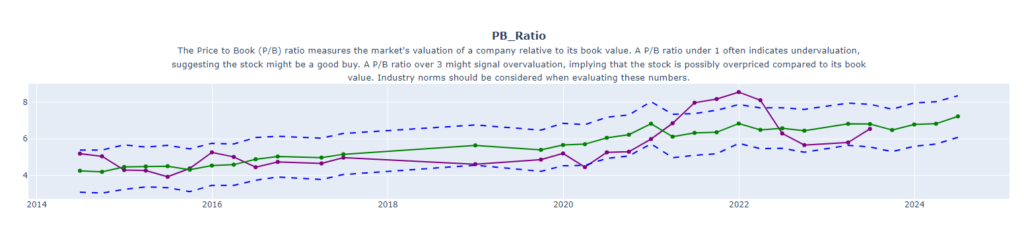
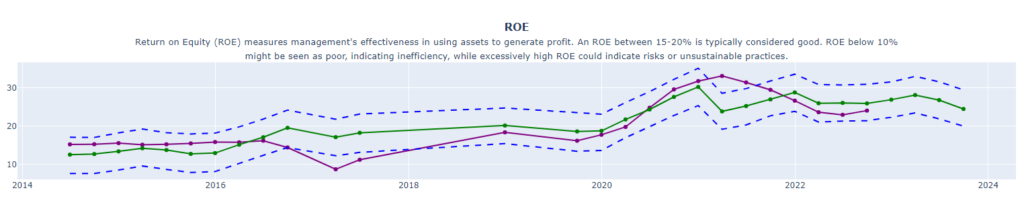



Financial ratios are key indicators of a company’s financial health and performance. They offer crucial insights into various aspects like profitability, liquidity, and valuation. In this section, we’ll leverage the predictive power of Facebook Prophet to forecast these ratios over the next year.
Forecasting Future Earnings and Profitability Metrics from the Income Statement with Facebook Prophet: A One-Year Outlook
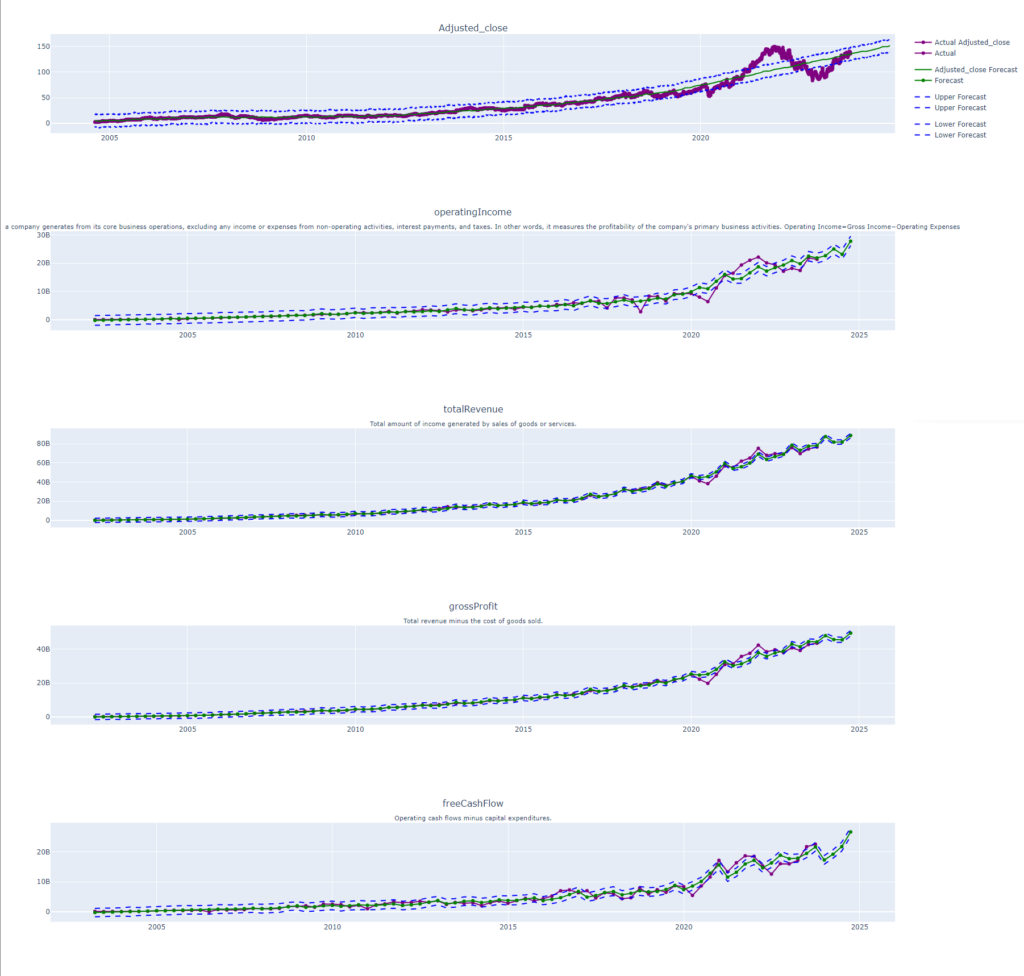
These plots present a comprehensive visualization of key earnings and profitability metrics extracted from the income statement, including Net Income, Operating Income, Total Revenue, Gross Profit, and Free Cash Flow. The financial numbers are then forward projected using Facebook Prophet. These predictions not only display the expected trends for the next year but also encompass upper and lower bounds, providing a range of possible outcomes. This integration of historical data with advanced forecasting techniques offers a nuanced understanding of the company’s financial trajectory.
Forecasting Future Balance Sheet Metrics with Facebook Prophet: A One-Year Outlook
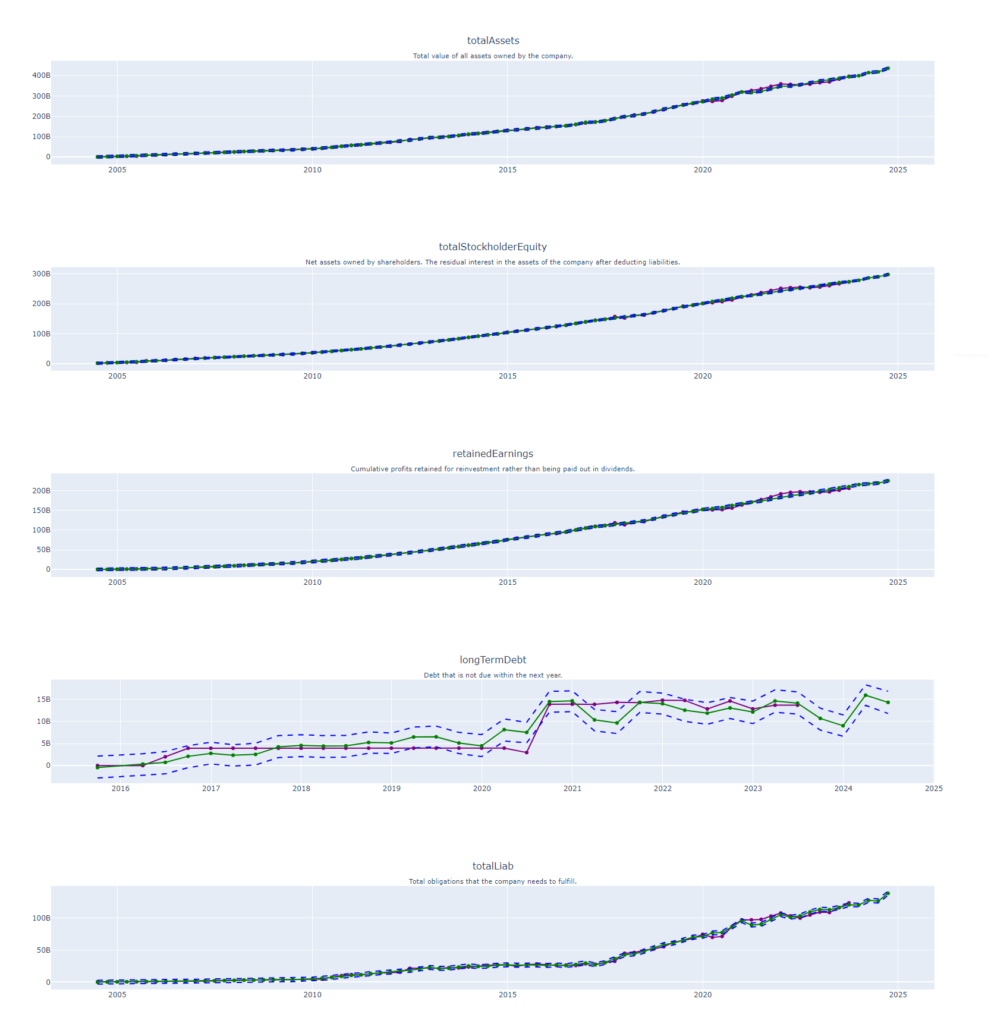
These plots offer an in-depth analysis of crucial balance sheet metrics such as Total Assets, Total Stockholder Equity, Retained Earnings, Long-Term Debt, and Total Liabilities. Leveraging the predictive power of Facebook Prophet, these key financial indicators are forecasted into the future. The projections not only illustrate the expected financial posture for the forthcoming year but also include upper and lower prediction intervals, giving a full spectrum of potential financial scenarios. By melding past balance sheet data with sophisticated predictive modeling, the plots provide a multi-dimensional view of the company’s expected financial stability and risk profile.
Forecasting Future Cash Flow Metrics with Facebook Prophet: A One-Year Outlook
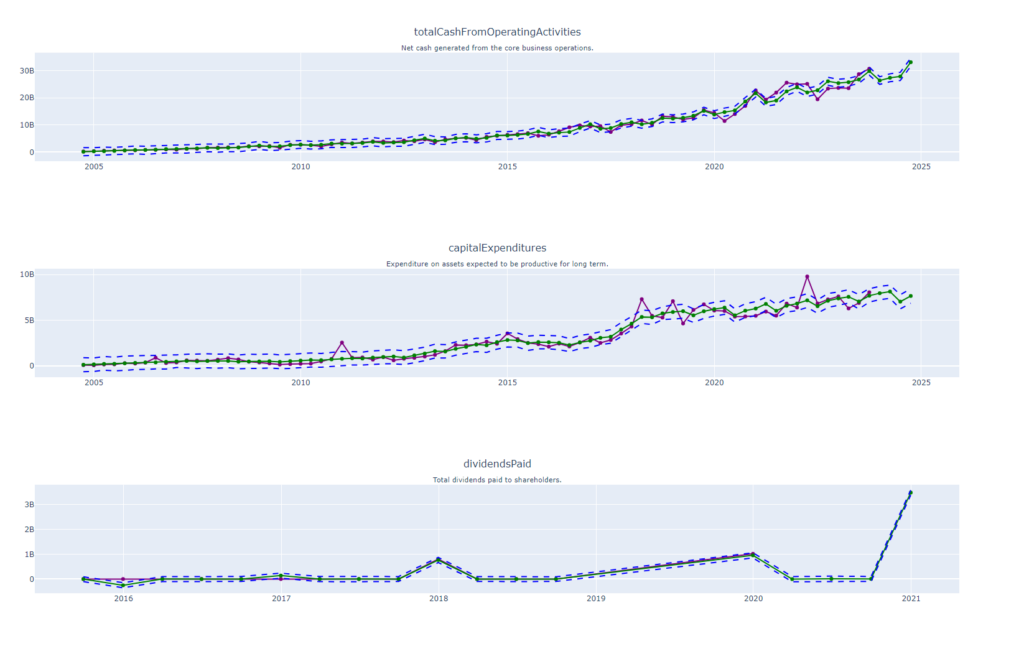
These plots dive into essential cash flow metrics, specifically Total Cash from Operating Activities, Capital Expenditures, and Dividends Paid. These key figures are also extended into the future using Facebook Prophet’s forecasting capabilities. The resulting predictions not only map out the anticipated cash flow movements for the next year but are also bracketed by upper and lower confidence intervals, presenting a comprehensive range of financial possibilities. These plots offer a well-rounded perspective on the company’s future liquidity and capital allocation strategies.
Other Financial Metrics
In addition to the key financial metrics, the analysis delves into a diverse set of other metrics, plotting each meticulously. These metrics are categorized as follows:
Efficiency and Activity Metrics from Income Statement:
- Research Development
- Selling General Administrative
Stockholder’s Equity and Capital Structure:
- Common Stock Shares Outstanding
Additional Important Metrics:
- Net Debt
- Net Receivables
- Inventory
- Accounts Payable
- Total Current Assets
- Total Current Liabilities
Less Critical Metrics:
- Income Before Tax
- Cost of Revenue
- Intangible Assets
- Earning Assets
- Other Current Assets
- Deferred Long-Term Liabilities
- Other Current Liabilities
- Common Stock
- Capital Stock
- Other Liabilities
- Goodwill
- Other Assets
- Cash
- Cash and Equivalents
- Current Deferred Revenue
- Short-Term Debt
- Short/Long-Term Debt
- Short/Long-Term Debt Total
- Other Stockholder Equity
- Property Plant Equipment
- Long-Term Investments
- Net Tangible Assets
- Short-Term Investments
Other Metrics:
- Effect of Accounting Charges
- Income Tax Expense
- Non-Operating Income Net Other
- Selling and Marketing Expenses
- Common Stock Total Equity
- Preferred Stock Total Equity
- Retained Earnings Total Equity
- Treasury Stock
- Accumulated Amortization
- Non-Current Assets Other
- Deferred Long-Term Asset Charges
- Non-Current Assets Total
- Capital Lease Obligations
- Long-Term Debt Total
- Non-Current Liabilities Other
- Non-Current Liabilities Total
- Negative Goodwill
- Warrants
- Preferred Stock Redeemable
- Capital Surpluse
- Liabilities And Stockholders Equity
- Cash And Short-Term Investments
- Property Plant And Equipment Gross
- Property Plant And Equipment Net
- Accumulated Depreciation
- Total Cash Flows From Investing Activities
- Total Cash From Financing Activities
- Net Borrowings
- Issuance Of Capital Stock
- Investments
- Change To Liabilities
- Change To Operating Activities
- Change In Cash
- Begin Period Cash Flow
- End Period Cash Flow
- Depreciation
- Other Cash Flows From Investing Activities
- Change To Inventory
- Change To Account Receivables
- Sale Purchase Of Stock
- Other Cash Flows From Financing Activities
- Change To Net Income
- Change Receivables
- Cash Flows Other Operating
- Exchange Rate Changes
- Cash And Cash Equivalents Changes
- Change In Working Capital
- Stock Based Compensation
- Other Non-Cash Items
Code Overview
Prerequisites
- Python 3.x
- Pandas
- Plotly
- Prophet
- Logging
- Pathlib
- os
- Collections
- bs4 (BeautifulSoup)
- time
- scikit-learn
Helper Functions
The code includes several helper functions, such as:
calculate_mae
: Calculates the Mean Absolute Error between the actual and predicted data.access_hdf5_with_retries
: Attempts to read an HDF5 file, retrying up to a specified number of times.read_general_info
: Reads general company information from an HDF5 file.infer_dtype
: Infers the data type of a Pandas Series.fetch_data_for_symbol_from_multiple_h5
: Fetches data for a specific symbol from multiple HDF5 files.get_company_name
: Gets the company name for a given symbol from an HDF5 file.add_content_before_plot
: Adds additional content before the plot in an HTML file.
Forecasting Function
The core of this code is the forecast_with_multiple_metrics
function, which:
- Accepts a DataFrame of financial metrics.
- Performs time-series forecasting on each metric using Facebook’s Prophet.
- Plots the actual and forecasted metrics using Plotly.
def forecast_with_multiple_metrics(df: pd.DataFrame, periods: int = 4, save_dir: str = "plots/", use_all_data: bool = True, eod_price_data: str = 'eod_price_data.h5'):
...
Code
import pandas as pd
from pathlib import Path
import logging
import os
from plotly.subplots import make_subplots
from prophet import Prophet
import plotly.graph_objects as go
from collections import Counter
from pandas.api.types import is_numeric_dtype
from bs4 import BeautifulSoup
import time
from sklearn.metrics import mean_absolute_error
from math import log10
from dictionaries_and_lists import metric_definitions, reordered_columns, homebuilders, sp500, companies_with_treasuries, largest_banks, percentage_metrics
data_dir = '/home/shared/algos/data/'
plots_dir = '/home/shared/algos/eodhd_data/plots/'
logging.basicConfig(level=logging.DEBUG)
logging.basicConfig(level=logging.INFO)
# Configure Pandas to display all columns
pd.set_option('display.max_columns', None)
pd.set_option('display.expand_frame_repr', False)
symbol_exchange_map = {}
def wrap_text(text, max_length):
"""
Wraps text to a new line at the nearest whitespace of the max_length.
"""
wrapped_lines = []
while len(text) > max_length:
# Find nearest whitespace of the max_length
split_index = text.rfind(' ', 0, max_length + 1)
if split_index == -1: # No whitespace found, force split
split_index = max_length
wrapped_lines.append(text[:split_index])
text = text[split_index:].lstrip()
wrapped_lines.append(text)
return '<br>'.join(wrapped_lines)
def format_large_number(num):
print('Attempting to plot large number')
if num < 1_000:
return str(num)
magnitude = int(log10(num) // 3)
value = num / (10 ** (3 * magnitude))
return f"{value:.2f}{' KMBT'[magnitude]}"
def format_percentage(data):
try:
# Remove any non-numeric characters like commas and percentage signs
if isinstance(data, str):
data = data.replace(',', '').replace('%', '').strip()
# Convert to float and format as a percentage
return "{:.2%}".format(float(data))
except ValueError as e:
print(f"ValueError: Could not convert {data} to a percentage.")
return data # Return the original data if it cannot be converted
def calculate_mae(actual, predicted):
return mean_absolute_error(actual, predicted)
def access_hdf5_with_retries(hdf5_file_path, mode, max_retries=3, sleep_duration=5):
retries = 0
while retries < max_retries:
try:
with pd.HDFStore(hdf5_file_path, mode) as store:
return store.keys() # You can customize this part to return what you need
break # If successful, exit the while loop
except Exception as e: # Replace Exception with a more specific exception if possible
if retries < max_retries - 1:
logging.info(f"An exception occurred while reading {hdf5_file_path}: {e}")
logging.info(f"Retrying in {sleep_duration} seconds...")
time.sleep(sleep_duration)
retries += 1
else:
logging.error(f"Max retries reached. Could not read {hdf5_file_path}. Exiting...")
raise
def read_general_info(symbol, h5_general_path, max_retries=3, sleep_duration=5):
retries = 0
while retries < max_retries:
try:
with pd.HDFStore(h5_general_path, 'r') as store:
key = f'/{symbol}'
if key in store.keys():
general_info = store.get(key)
info_dict = dict(zip(general_info['SubCategory'], general_info['Data']))
sector = info_dict.get('Sector', 'N/A')
industry = info_dict.get('Industry', 'N/A')
description = info_dict.get('Description', 'N/A')
full_time_employees = info_dict.get('FullTimeEmployees', 'N/A')
updated_at = info_dict.get('UpdatedAt', 'N/A')
web_url = info_dict.get('WebURL', 'N/A')
phone = info_dict.get('Phone', 'N/A')
address = info_dict.get('Address', 'N/A')
name = info_dict.get('Name', 'N/A')
exchange = info_dict.get('Exchange', 'N/A')
return sector, industry, description, full_time_employees, updated_at, web_url, phone, address, name, exchange
else:
return ['N/A']*10
break
except Exception as e:
if retries < max_retries - 1:
print(f"An exception occurred while reading {h5_general_path}: {e}")
print(f"Retrying in {sleep_duration} seconds...")
time.sleep(sleep_duration)
retries += 1
else:
print(f"Max retries reached. Could not read {h5_general_path}. Exiting...")
raise
def infer_dtype(series):
sample = series.dropna().head(100) # Sample the first 100 non-null rows
# Debugging line
print(f"Sample for {series.name}: {sample}")
if sample.empty:
return None
if all(sample.apply(lambda x: isinstance(x, str))):
return 'object'
if is_numeric_dtype(sample) and all(sample.apply(lambda x: x == int(x))):
return 'int64'
if is_numeric_dtype(sample):
return 'float64'
return None
def fetch_data_for_symbol_from_multiple_h5(h5_filepaths, symbol, max_retries=3, sleep_duration=5):
combined_data = None
common_keys = ['Symbol', 'date', 'filing_date', 'currency_symbol']
for h5_filepath in h5_filepaths:
h5_path = Path(h5_filepath)
if not h5_path.exists():
logging.info(f"The file {h5_filepath} does not exist.")
continue
retries = 0
while retries < max_retries:
try:
with pd.HDFStore(h5_filepath, 'r') as store:
if f"/{symbol}" in store.keys():
logging.info(f"Symbol {symbol} found in {h5_filepath}.")
symbol_data = store.select(symbol)
if 'date' not in symbol_data.columns and 'Date' not in symbol_data.columns:
if isinstance(symbol_data.index, pd.DatetimeIndex):
symbol_data.reset_index(inplace=True)
symbol_data.rename(columns={'index': 'date'}, inplace=True)
logging.info(
f"'date' and 'Date' columns not found, but datetime index exists in {h5_filepath} for symbol {symbol}.")
symbol_data = symbol_data.reset_index().rename(columns={'index': 'date'})
else:
logging.warning(
f"'date' and 'Date' columns and datetime index not found in {h5_filepath} for symbol {symbol}.")
break
else:
# Ensure the date column is standardized to 'date'
if 'Date' in symbol_data.columns:
symbol_data.rename(columns={'Date': 'date'}, inplace=True)
# Rename 'netIncome' if it's present in the dataframe
if 'netIncome' in symbol_data.columns:
# Extract the type of financial statement from the filename
parts = h5_path.stem.split('_')
statement_type = parts[1]
metric_name = parts[2]
statement_readable = {
"Cash": "Cash Flow from Operating Activities",
"Income": "netIncome",
}.get(statement_type, statement_type)
metric_title = f"{metric_name} ({statement_readable})"
# Ensure statement_type is one of the reports where 'netIncome' appears before renaming
if statement_type in ["Income", "Cash"]:
symbol_data.rename(columns={'netIncome': metric_title}, inplace=True)
if combined_data is None:
combined_data = symbol_data
else:
# Dynamically adjust common keys based on available columns
keys_for_merge = [key for key in common_keys if
key in symbol_data.columns and key in combined_data.columns]
combined_data = pd.merge(combined_data, symbol_data, on=keys_for_merge, how='outer')
break
else:
logging.info(f"Symbol {symbol} not found in {h5_filepath}.")
break
except Exception as e:
if retries < max_retries - 1:
logging.info(f"An exception occurred while reading {h5_filepath}: {e}")
logging.info(f"Retrying in {sleep_duration} seconds...")
time.sleep(sleep_duration)
retries += 1
else:
logging.info(f"Max retries reached. Could not read {h5_filepath}. Exiting...")
raise
if combined_data is None:
logging.info(f"Symbol {symbol} not found in any of the HDF5 files.")
return None
else:
for col in combined_data.select_dtypes(include=['object']).columns:
combined_data[col] = pd.to_numeric(combined_data[col], errors='ignore')
if 'date' in combined_data.columns:
combined_data.sort_values(by='date', inplace=True)
else:
logging.info(f"'date' column not found in combined data for symbol {symbol}. Sorting by index instead.")
combined_data.sort_index(inplace=True)
logging.info(f"{symbol}: successfully combined all HDF5 files.")
return combined_data
def get_company_name(symbol, h5_file_path, country_code='US'):
try:
# Read the DataFrame for the entire country from the HDF5 file
symbols_df = pd.read_hdf(h5_file_path, key=f'/{country_code}')
# Filter by the specific symbol to get the company name
company_name_row = symbols_df[symbols_df['Code'] == symbol]
if not company_name_row.empty:
return company_name_row['Name'].iloc[0]
else:
logging.error(f"No data for symbol {symbol} in the DataFrame.")
return "Unknown"
except KeyError:
logging.error(f"No object named {country_code} in the file {h5_file_path}")
return "Unknown"
def add_content_before_plot(symbol, max_retries=3, sleep_duration=5):
target_filename = f"{symbol}_quarterly.html"
html_file_path = os.path.join(plots_dir, target_filename)
if not os.path.exists(html_file_path):
print(f"HTML file for symbol {symbol} not found.")
return
# Fetch additional info from General.h5
exchange = symbol_exchange_map.get(symbol, 'Other') # Default to 'Other' if exchange is not found
h5_general_path = os.path.join(data_dir, f"{exchange}_General.h5")
sector, industry, description, full_time_employees, updated_at, web_url, phone, address, name, exchange = read_general_info(symbol, h5_general_path, max_retries, sleep_duration)
# Prepare new HTML content
new_html_content = f"""
<h1>{symbol} - {name} - {exchange}</h1>
<p>Updated At: {updated_at}</p>
<p><strong style='font-size: larger;'>WebURL:</strong> <a href='{web_url}' target='_blank'>{web_url}</a>
<strong style='font-size: larger;'>Phone:</strong> {phone}
<strong style='font-size: larger;'>Address:</strong> {address}<br>
<strong style='font-size: larger;'>Sector:</strong> {sector}
<strong style='font-size: larger;'>Industry:</strong> {industry}<br>
<strong style='font-size: larger;'>Full Time Employees:</strong> {full_time_employees}</p><br>
<strong style='font-size: larger;'>Description:</strong> {description}<br><br>
"""
# Read valuation.h5 data for the symbol
h5_valuation_path = os.path.join(data_dir, f"{exchange}_Valuation.h5")
retries = 0
valuation_data = None
while retries < max_retries:
try:
with pd.HDFStore(h5_valuation_path, 'r') as store:
if symbol in store:
valuation_data = store.get(symbol)
else:
valuation_data = pd.DataFrame(columns=['SubCategory', 'Data'])
print(f"The symbol {symbol} valuation data does not exist in {h5_valuation_path}")
break
except Exception as e:
if retries < max_retries - 1:
print(f"An exception occurred while reading {h5_valuation_path}: {e}")
print(f"Retrying in {sleep_duration} seconds...")
time.sleep(sleep_duration)
retries += 1
else:
print(f"Max retries reached. Could not read {h5_valuation_path}. Exiting...")
raise
# Add valuation_data to new_html_content
valuation_html = "<h2>Valuation</h2><div style='display: flex; flex-wrap: wrap;'>"
if valuation_data is not None:
print(f"valuation_data: {valuation_data}")
valuation_data['Data'] = pd.to_numeric(valuation_data['Data'], errors='coerce')
for index, row in valuation_data.iterrows():
data = row['Data']
if pd.notnull(data): # Check if 'Data' is not NaN
if index in percentage_metrics:
formatted_data = "{:.2%}".format(float(data))
elif isinstance(data, (int, float)) and abs(data) >= 1_000: # Large numbers
formatted_data = format_large_number(data)
elif isinstance(data, (int, float)):
print('formatting numbers with commas in Valuation')
print(f"data: {data}, type: {type(data)}")
formatted_data = f"{data:,.2f}" # For other numbers, just format with commas
else:
formatted_data = data # For non-numeric data, leave as is
else:
# If data is NaN or non-numeric, leave as is
formatted_data = row['Data']
valuation_html += f"<div style='flex: 0 0 calc(33.333% - 10px); margin-right: 10px; margin-bottom: 10px;'>"
valuation_html += f"<strong>{row['SubCategory']}</strong>: {formatted_data}</div>"
valuation_html += "</div>"
new_html_content += valuation_html # Append valuation data to new_html_content
# Read highlights.h5 data for the symbol
h5_highlights_path = os.path.join(data_dir, f"{exchange}_Highlights.h5")
retries = 0
while retries < max_retries:
try:
with pd.HDFStore(h5_highlights_path, 'r') as store:
if symbol in store:
highlights_data = store.get(symbol)
else:
highlights_data = pd.DataFrame(columns=['SubCategory', 'Data'])
print(f"The symbol {symbol} highlights data does not exist in {h5_highlights_path}")
break
except Exception as e:
if retries < max_retries - 1:
print(f"An exception occurred while reading {h5_highlights_path}: {e}")
print(f"Retrying in {sleep_duration} seconds...")
time.sleep(sleep_duration)
retries += 1
else:
print(f"Max retries reached. Could not read {h5_highlights_path}. Exiting...")
raise
# Add title before highlights
new_html_content += "<h2>Highlights</h2>"
# Add highlights_data to new_html_content
highlights_html = "<div style='display: flex; flex-wrap: wrap;'>"
highlights_data['Data'] = highlights_data['Data'].apply(pd.to_numeric, errors='ignore')
for index, row in highlights_data.iterrows():
subcategory = row['SubCategory']
data = row['Data']
if subcategory in percentage_metrics:
formatted_data = format_percentage(data)
elif pd.notnull(data) and isinstance(data, (int, float)):
if abs(data) >= 1_000: # Large numbers
print(f"{symbol}: {subcategory} is a large number. Formatting {data} with commas...")
formatted_data = format_large_number(data)
else: # Other numeric data that is not a large number
print(f"{symbol}: {subcategory} is a numeric value. Formatting {data} with commas...")
formatted_data = f"{data:,.2f}"
else:
print(f'{symbol}: {subcategory} is a non-numeric value. Data: {data}')
formatted_data = data # For non-numeric data, leave as is
highlights_html += f"""<div style='flex: 0 0 calc(33.333% - 10px); margin-right: 10px; margin-bottom: 10px;'>
<strong>{subcategory}</strong>: {formatted_data}
</div>"""
highlights_html += "</div>"
new_html_content += highlights_html # App
# Add the disclaimer to the end of new_html_content
disclaimer = "<br><br><p><strong>Disclaimer:</strong> The last data point has been excluded from the Prophet prediction.</p>"
new_html_content += disclaimer
with open(html_file_path, 'r', encoding='utf-8') as f:
html_content = f.read()
# Parse the HTML content with BeautifulSoup
soup = BeautifulSoup(html_content, 'html.parser')
# Find the div that contains the Plotly plot
plot_div = soup.find('div', {'class': 'plotly-graph-div'})
if plot_div:
# Create a BeautifulSoup object from the new_content string
new_content_soup = BeautifulSoup(new_html_content, 'html.parser')
# Insert the new content before the Plotly plot
plot_div.insert_before(new_content_soup)
# Save the modified HTML back to disk
with open(html_file_path, 'w', encoding='utf-8') as f:
f.write(str(soup))
else:
print(f"Plotly plot not found in the HTML file {html_file_path}.")
def forecast_with_multiple_metrics(df: pd.DataFrame, periods: int = 4, save_dir: str = "plots/", use_all_data: bool = True, eod_price_data: str = 'eod_price_data.h5'):
def plot_adjusted_close_from_h5(fig, symbol, hdf5_file_path, max_retries=3, sleep_duration=5):
hdf5_file_path = data_dir + hdf5_file_path
keys = access_hdf5_with_retries(hdf5_file_path, 'r', max_retries, sleep_duration)
if f'/{symbol}' in keys:
with pd.HDFStore(hdf5_file_path, 'r') as store:
stock_data = store.get(symbol)
# Add actual adjusted_close to the plot
fig.add_trace(
go.Scatter(x=stock_data.index, y=stock_data['Adjusted_close'],
mode='lines+markers',
name='Actual Adjusted_close',
legendgroup='Actual',
line=dict(color='purple'),
showlegend=True),
row=1, col=1
)
prophet_df = stock_data.reset_index()[['Date', 'Adjusted_close']].rename(
columns={'Date': 'ds', 'Adjusted_close': 'y'})
best_mae = float('inf')
best_forecast = None
best_mode = None
for mode in ['additive', 'multiplicative']:
model = Prophet(
seasonality_mode=mode,
yearly_seasonality=True,
weekly_seasonality=False,
daily_seasonality=False)
model.fit(prophet_df)
future = model.make_future_dataframe(periods=365) # 1-year prediction
forecast = model.predict(future)
mae = calculate_mae(prophet_df['y'], forecast.loc[:len(prophet_df) - 1, 'yhat'])
if mae < best_mae:
best_mae = mae
best_forecast = forecast
best_mode = mode
# Add the best forecast to the plot
fig.add_trace(
go.Scatter(x=best_forecast['ds'], y=best_forecast['yhat'],
mode='lines',
name='Adjusted_close Forecast',
legendgroup='Forecast',
line=dict(color='green'),
showlegend=True),
row=1, col=1
)
# Add yhat_upper and yhat_lower to the plot
fig.add_trace(
go.Scatter(x=best_forecast['ds'], y=best_forecast['yhat_upper'],
mode='lines',
name='Upper Forecast',
legendgroup='Upper Forecast',
line=dict(color='blue', dash='dash'),
showlegend=True),
row=1, col=1
)
fig.add_trace(
go.Scatter(x=best_forecast['ds'], y=best_forecast['yhat_lower'],
mode='lines',
name='Lower Forecast',
legendgroup='Lower Forecast',
line=dict(color='blue', dash='dash'),
showlegend=True),
row=1, col=1
)
else:
logging.warning(f"No Adjusted_close data found for symbol: {symbol}")
pd.set_option('display.max_columns', None)
logging.debug(df.columns.tolist())
# Get the columns that are both in df.columns and reordered_columns
common_columns = [col for col in reordered_columns if col in df.columns]
# Check if common_columns is not empty
if common_columns:
# Reorder the DataFrame using the common columns
df = df[common_columns + [col for col in df.columns if col not in common_columns]]
else:
logging.warning("No common columns between df and reordered_columns.")
metrics = [col for col in df.columns if df[col].dtype in ['int64', 'float64']]
logging.info(metrics)
subplot_titles = []
for metric in metrics:
definition = metric_definitions.get(metric, 'No definition available')
wrapped_definition = wrap_text(definition, 160) # Adjust the max_length as needed
title = f"<b>{metric}</b><br><span style='font-size: smaller;'>{wrapped_definition}</span>"
subplot_titles.append(title)
fig = make_subplots(rows=len(subplot_titles) + 1, cols=1, subplot_titles=['Adjusted_close'] + subplot_titles)
symbol = df['Symbol'].iloc[0]
plot_adjusted_close_from_h5(fig, symbol, eod_price_data)
# Fetch the company_name
h5_symbols = os.path.join(data_dir, "symbols.h5")
company_name = get_company_name(symbol, h5_symbols)
if not os.path.exists(save_dir):
os.makedirs(save_dir)
if not use_all_data:
disclaimer = "Disclaimer: The last data point has been excluded from the Prophet prediction. \n "
disclaimer += "There may not be enough data points for Prophet to make accurate predictions. \n"
fig.add_annotation(
dict(
x=0,
y=1.1,
xref="paper",
yref="paper",
text=disclaimer,
showarrow=False,
font=dict(size=16)
)
)
for i, metric in enumerate(metrics):
row = i + 2
plotting_df = df[['date', metric]].copy().dropna()
prophet_df = plotting_df.copy()
# Convert the specified metrics to percentages by multiplying by 100
if metric in ['ROE', 'Earnings_Yield', 'Dividend_Yield']:
prophet_df[metric] = prophet_df[metric] * 100 # Convert to percentage
plotting_df[metric] = plotting_df[metric] * 100 # Convert to percentage
if not use_all_data:
prophet_df = prophet_df.iloc[:-1, :]
prophet_df.rename(columns={'date': 'ds', metric: 'y'}, inplace=True)
if prophet_df.shape[0] < 2:
logging.warning(f"Skipping {metric} because it has less than 2 non-NaN rows.")
continue
best_mae = float('inf')
best_forecast = None
best_mode = None
for mode in ['additive', 'multiplicative']:
model = Prophet(
seasonality_mode=mode,
yearly_seasonality=True,
weekly_seasonality=False,
daily_seasonality=False)
model.fit(prophet_df)
future = model.make_future_dataframe(periods=periods, freq='Q')
forecast = model.predict(future)
mae = calculate_mae(prophet_df['y'], forecast.loc[:len(prophet_df) - 1, 'yhat'])
if mae < best_mae:
best_mae = mae
best_forecast = forecast
best_mode = mode
logging.info(f"Best seasonality mode for {metric} is {best_mode} with MAE {best_mae}")
hover_format = '%{x}: %{y:.2f}%' if metric in ['ROE', 'Earnings_Yield', 'Dividend_Yield'] else '%{x}: %{y:,}'
fig.add_trace(
go.Scatter(x=plotting_df['date'], y=plotting_df[metric],
mode='lines+markers',
name=f'Actual {metric}',
legendgroup='Actual',
line=dict(color='purple'),
showlegend=(i == 0),
hovertemplate=hover_format),
row=row, col=1
)
# Plot the forecasted metric data
fig.add_trace(
go.Scatter(x=best_forecast['ds'],
y=best_forecast['yhat'],
mode='lines+markers',
name=f'Forecasted {metric}',
legendgroup='Forecast',
line=dict(color='green'),
showlegend=(i == 0),
hovertemplate=hover_format),
row=row, col=1
)
# Plot the upper forecast interval
fig.add_trace(
go.Scatter(x=best_forecast['ds'], y=best_forecast['yhat_upper'],
mode='lines',
name=f'Upper Bound {metric}',
legendgroup='Upper Forecast',
line=dict(color='blue', dash='dash'),
showlegend=(i == 0),
hovertemplate=hover_format),
row=row, col=1
)
# Plot the lower forecast interval
fig.add_trace(
go.Scatter(x=best_forecast['ds'], y=best_forecast['yhat_lower'],
mode='lines',
name=f'Lower Bound {metric}',
legendgroup='Lower Forecast',
line=dict(color='blue', dash='dash'),
showlegend=(i == 0),
hovertemplate=hover_format),
row=row, col=1
)
fig.update_layout(
height=350 * len(metrics),
width=1800,
title_font_size=16 # You can change this value as needed
)
plot_file_path = os.path.join(save_dir, f"{symbol}_quarterly.html")
fig.write_html(plot_file_path)
logging.info(f"Saved Prophet plot for {company_name} to {plot_file_path}")
add_content_before_plot(symbol)
def get_symbols(h5_file_path, key='US'):
"""
Open an HDF5 file and populate the global dictionary symbol_exchange_map
where the symbol is the key and the exchange is the value.
Parameters:
h5_file_path (str): The path to the HDF5 file.
key (str): The key to use when reading the HDF5 file. Default is 'US'.
Returns:
None
"""
h5_file_path = Path(h5_file_path)
# Check if the file exists
if not h5_file_path.exists():
logging.info(f"The file {h5_file_path} does not exist.")
return
try:
# Read the DataFrame from the HDF5 file
df = pd.read_hdf(h5_file_path, key=key)
# Check if 'Code' and 'Exchange' columns exist
if 'Code' not in df.columns or 'Exchange' not in df.columns:
logging.info(f"The 'Code' or 'Exchange' column does not exist in the DataFrame.")
return
# Populate the global symbol_exchange_map
global symbol_exchange_map
symbol_exchange_map = dict(zip(df['Code'], df['Exchange']))
return list(symbol_exchange_map.keys())
except Exception as e:
logging.error(f"An error occurred: {e}")
return
symbols = get_symbols(data_dir + 'symbols.h5', key='US')
symbols = ['UBER', 'LYFT', 'WE', 'IEP', 'AAPL'] + sp500 + largest_banks + companies_with_treasuries + homebuilders
symbols = ['GDHG', 'IEP']
symbols = ['GOOG']
h5_files = set() # Use a set to automatically handle duplicates
for symbol in symbols:
exchange = symbol_exchange_map.get(symbol, 'Other') # Default to 'Other' if not found
current_h5_files = [
f"{data_dir}/{exchange}_Cash_Flow_quarterly.h5",
f"{data_dir}/{exchange}_Balance_Sheet_quarterly.h5",
f"{data_dir}/{exchange}_Income_Statement_quarterly.h5",
f"{data_dir}/{exchange}_Ratios.h5"
]
h5_files.update(current_h5_files) # Update the set with the new file paths
logging.info(f'Processing {symbol}')
try:
# Pass only the HDF5 files corresponding to the current symbol's exchange
df = fetch_data_for_symbol_from_multiple_h5(list(current_h5_files), symbol, max_retries=3, sleep_duration=5)
if df is None:
logging.warning(f"No data found for symbol {symbol}. Skipping to the next symbol.")
continue # Skip to the next iteration of the loop forecast_with_multiple_metrics(df, periods=4, save_dir="plots/", use_all_data=False)
logging.info(df.head(20))
forecast_with_multiple_metrics(df)
except Exception as e:
logging.error(f"An unexpected error occurred while processing symbol {symbol}: {e}")